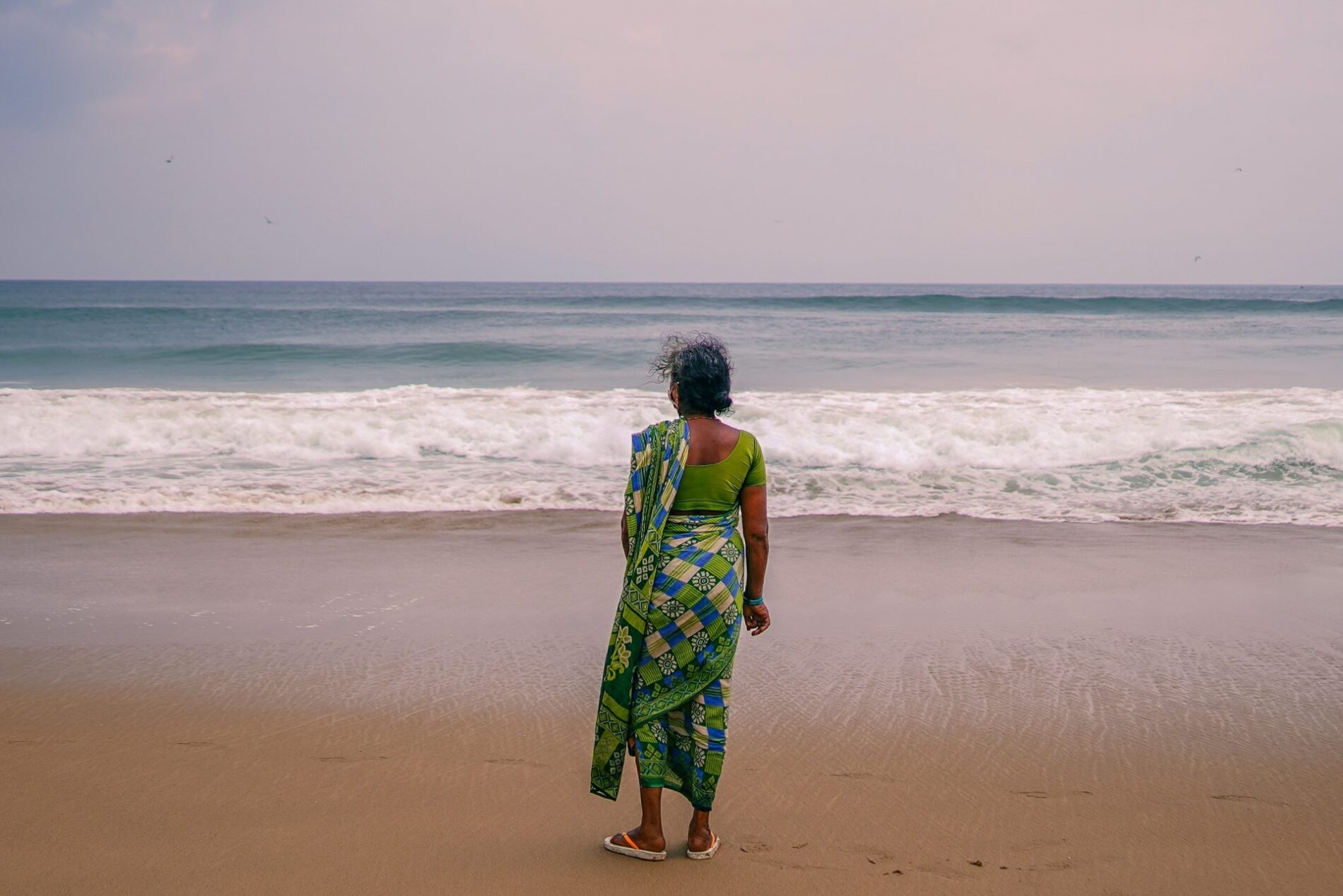
This non-profit is protecting vulnerable communities from the effects of climate change with AI
Armed with Microsoft’s AI for Humanitarian Action grant, SEEDS is using AI to save lives during disasters
“We fled to the nearby forest and clung on to swaying trees for our lives. We prayed for the storm to pass, but we lost everything we had,” says Kalpreddy Satyavati, who runs a tiny food stall catering to the local fishing community in the coastal town of Puri.
She was among the lucky ones who survived a “super cyclone” that tore through the state of Odisha on India’s east coast in 1999. Winds of up to 160 miles an hour (257 kilometers an hour) killed thousands and left behind a trail of destruction. Cyclones–known elsewhere as hurricanes and typhoons–are intense tropical storms that originate in the Indian Ocean.
But in May this year, when Cyclone Yaas was about to make landfall, things were a little different.
Satyavati received a personalized advisory printed in her language a day before the cyclone made landfall. The advisory, which she and nearly a thousand other families received, was the result of an artificial intelligence (AI) model by SEEDS, a non-profit that had recently received a Microsoft AI for Humanitarian Action grant.
Following the instructions on the sheet of paper, her family packed their belongings and left for a nearby cyclone shelter, one of the hundreds created after the 1999 super-cyclone. She even managed to move the contents of her food stall to a neighbor’s concrete house that could withstand the high-velocity winds.
Just like the advisory suggested, her rudimentary hut was destroyed, but she could resume her food stall immediately.
“Had we not received the advisory, it’d have been impossible for us to rebuild our lives,” says Satyavati, who lost her husband to COVID-19 last December.
As an AI for Humanitarian Action grant winner, SEEDS received financial and technical support from Microsoft and its technology partner Gramener to build an intuitive model that can forecast the impact of disasters on the most vulnerable populations.
“We usually come to know a cyclone’s predicted path 48 hours or so before it makes landfall. Manually collecting information about houses that’d be vulnerable is a very time-intensive task and we can, at best, evaluate 100 houses in a day,” says Raghav Ranganathan, technical director, SEEDS. “We started conversations with Microsoft to see if we could use AI to identify these houses so we could spend more time on the ground helping people.”
SEEDS stands for the Sustainable Environment and Ecological Development Society. It was founded in 1994 and works with vulnerable communities to provide relief and rehabilitation after disasters.
Microsoft launched AI for Humanitarian Action in 2018. It is a global grant program designed to help find solutions to challenges centered around disaster response, refugees, displaced people, human rights, and the needs of women and children.
The AI model, called Sunny Lives, utilizes high-resolution satellite imagery of areas likely to fall under the cyclone’s path. It then applies advanced data analytics and machine learning to identify the most vulnerable houses. This enables SEEDS and its on-ground partners to pinpoint those who’d be at the highest risk from the cyclone and focus their outreach to those communities.
According to the United Nations, the global mean sea level has risen faster since 1900, than over any preceding century in at least the last 3,000 years. A report by the Intergovernmental Panel on Climate Change (IPCC), released in August this year, declared a “code red” for humanity. It found that extreme weather conditions like droughts, hurricanes, and heatwaves have intensified because of climate change.
Satyavati doesn’t need any report to convince her about the impact of climate change on her community.
“When I was a child, I had to walk for nearly a mile to reach the beach. But the sea has now moved closer to our homes,” she says in her native Telugu language.
She shares her home—a hut made of bamboo and mud with palm leaves substituting for a roof—with her three daughters. The sea, while a source of income for her, is also a source of constant worry.
So far, she’s depended on good fortune and an overabundance of caution to protect her family from the tropical storms. Sometimes, however, good fortune is not enough. In November 2020, she lost all her belongings to Cyclone Nivar. Her house was destroyed, but it was the loss of her food stall that hit her the hardest.
“We lost all our wares to the storm and had no other means of livelihood. For days, we depended on the goodwill of others for food and survival,” she says.
Every roof tells a story
While state and local governments issue advisories in the event of an impending cyclone, they contain mostly generic information and cover entire districts.
SEEDS wanted to use AI to generate house-level advisories for communities at risk, so it could warn those who had the most to lose.
To build the AI model, they had to know who those people would be, and how they’d be impacted. The team had to find some attributes that could act as a proxy for the AI model to identify homes that would be vulnerable.
The SEEDS team quickly zeroed in on roof types.
“Roofs are a proxy of judging how vulnerable a building is. So, it became the first layer for us to build this model,” says Mridula Garg, an architect and urban researcher who’s working on the project.
“More importantly, the roofs also tell a lot about who lives inside and their socio-economic condition,” adds Ranganathan.
The logic is simple: a house with a reinforced cement concrete roof wouldn’t be as impacted by a tropical storm but a thatched roof, like the one Satayvati lives in, wouldn’t stand a chance.
If the AI model can identify houses with roofs that can’t withstand cyclonic wind speeds, the on-ground team can prioritize those households. But creating a model like this was easier said than done.
AI to the rescue
“We didn’t have another instance of AI being used to tag roof types to forecast damage due to hurricanes. In addition, there was no readily available training data,” says Tina Sederholm, a senior program manager in the AI for Good Research Lab at Microsoft, who led the project with data scientists.
“From a technical standpoint too, it was difficult because there is no urban planning in areas that we were targeting, and the population was so dense that it was difficult to first differentiate individual houses and categorize them accurately based on their roof type. But we built a machine learning model to counter these problems,” explains Md Nasir, a data scientist and researcher in the AI for Good Research Lab.
To create the much-needed training data, Gramener, with its expertise in geospatial solutions, stepped in to deliver a scalable solution. Its data scientists accessed high resolution satellite imagery and manually tagged more than 50,000 houses to classify their roofs under seven categories depending on the material used to construct them.
“We wanted to identify the building footprint and distinguish between two houses distinctly. But informal settlements do not often have well defined boundaries and they are generally the worst impacted in any disaster,” says Sumedh Ghatage, a data scientist from Gramener, who worked on building the AI model. “Secondly, as the geographical location changes, the types of roofs change as well. But we wanted to identify all kinds of roofs, to ensure the final model could be deployed in any region.”
This formed the basis of the training data Nasir required. After trying a few different techniques, his final model could identify roofs with an accuracy of nearly 90%. But that was just the beginning.
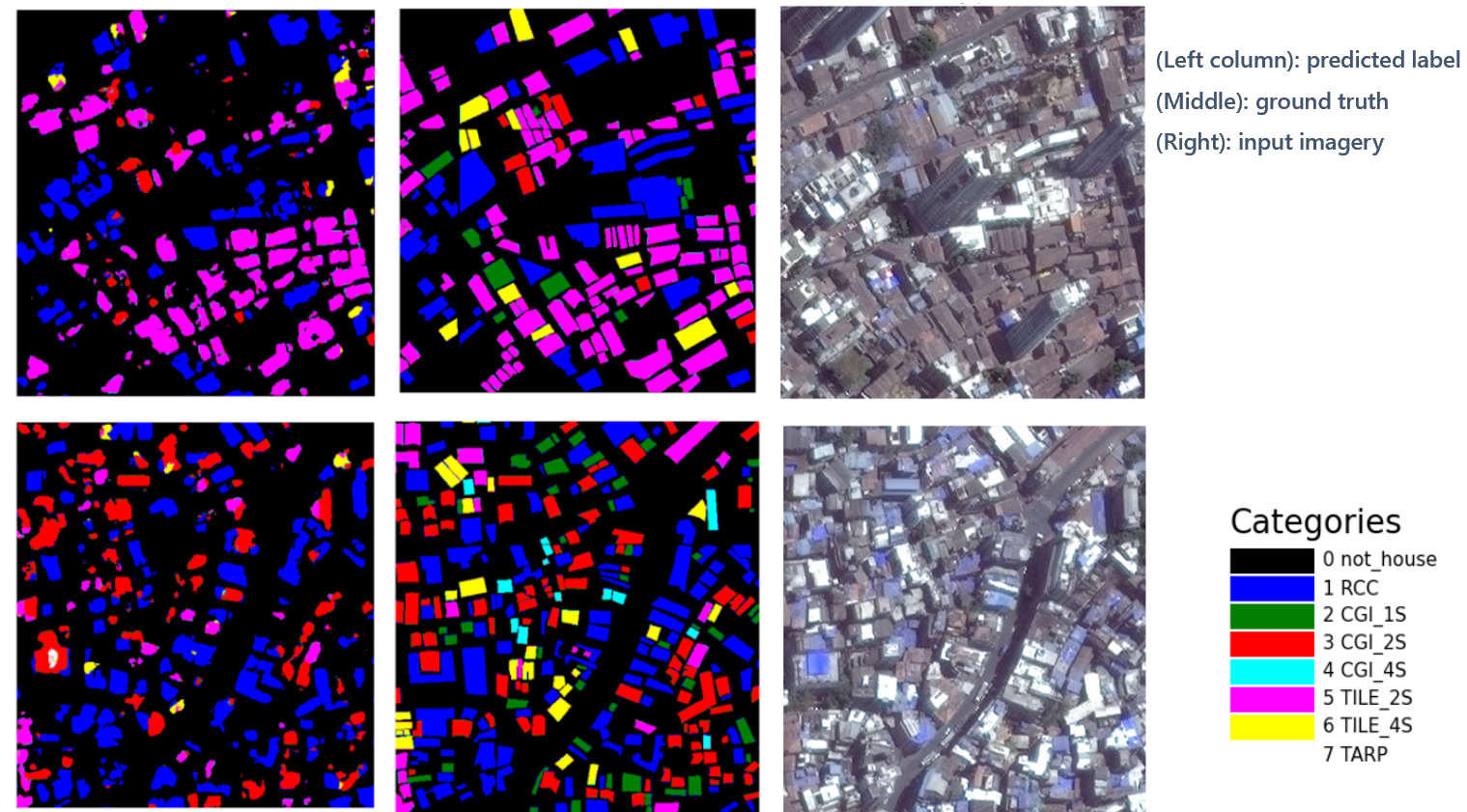
“Apart from roofs, we considered nearly a dozen critical parameters that determine the overall impact cyclones would have on a house,” says Kaustubh Jagtap from Gramener, who led the data consulting bits for the project. “For example, if a house is closer to a water body, it would be more likely to be impacted due to a cyclone-induced flood. Or if the area around the house is covered by concrete, the water won’t percolate into the soil below and odds of water logging and flooding would be higher.”
The team at Gramener then added other layers to the model. The alignment of all the different layers including road networks, proximity to water bodies, elevation profiles, vegetation, among others was a tedious task. Gramener created an Azure machine learning pipeline, which automatically captures the data and produces risk score profiles for every house.
It took about four months for the Sunny Lives model to become a reality and it was piloted during cyclones that hit southern Indian states of Tamil Nadu and Kerala in 2020. But it was during Cyclone Yaas in May this year that it was deployed at scale.
As soon as the path of Cyclone Yaas was predicted, the team at Gramener procured high resolution satellite imagery of densely populated areas that’d be impacted and ran the Sunny Lives AI model. In a few hours, they were able to create a risk score for every house in the area.
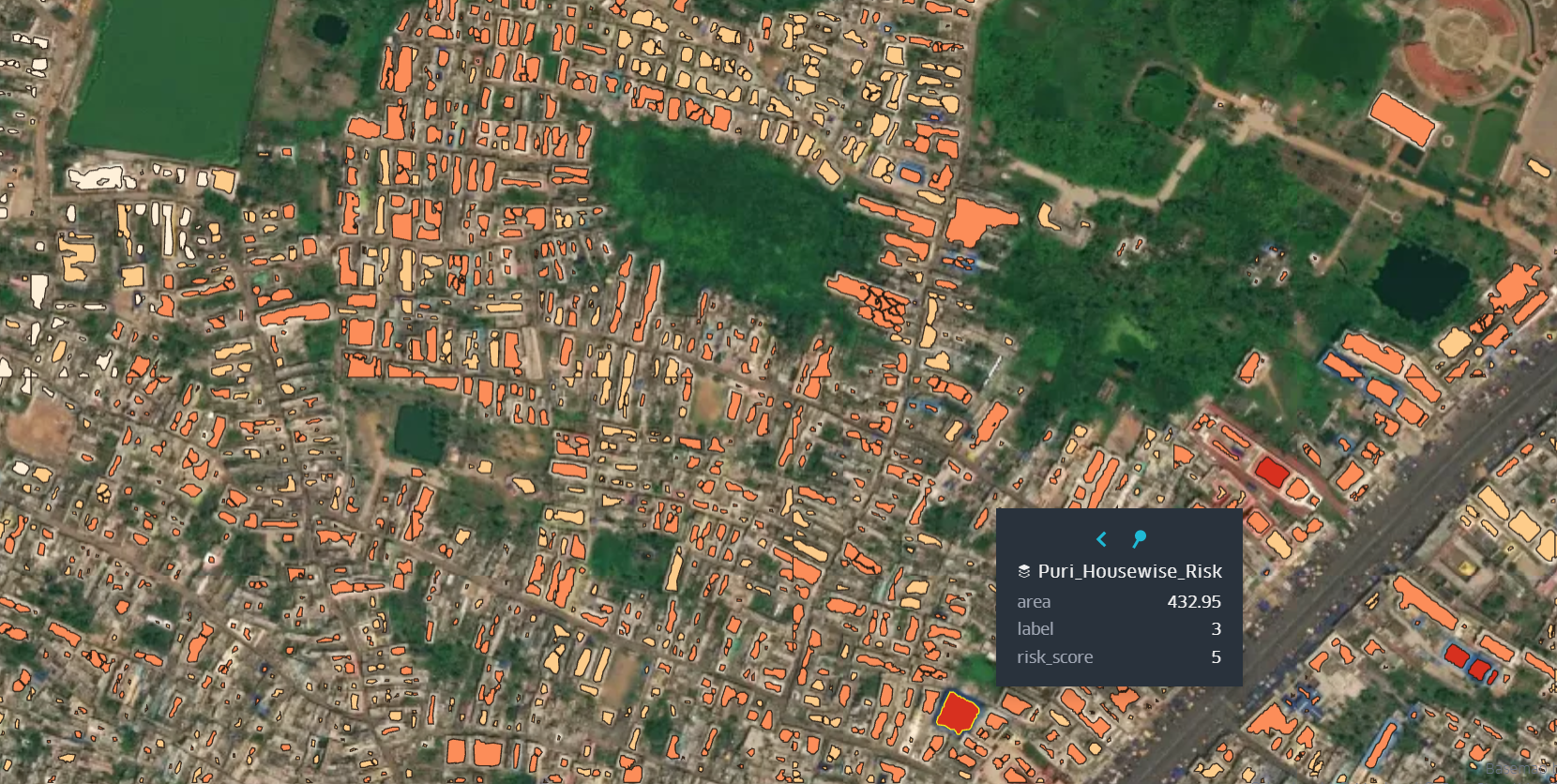
Gramener also assisted in sampling techniques and validated the accuracy of the model with actual ground truth information.
“Earlier, we used to deploy volunteers who manually conducted surveys. Now, all we need to do is procure high-resolution satellite imagery, run the model to determine an area’s vulnerability and get the risk score results within a day. This kind of capacity was unthinkable earlier,” says Garg.
Once the houses were identified, SEEDS along with its on-ground partners fanned out into the communities and distributed advisories to nearly 1,000 families in local languages like Telugu and Odia, which is spoken by the residents. Each advisory had detailed instructions on how they could secure their homes and where they would need to relocate to before the cyclone made landfall.
The model has opened a world of possibilities. SEEDS believes it can be deployed in many countries in Southeast Asia that share similar dwellings and communities that face the extreme levels of storm risk.
It can also be used to cope other weather challenges. For instance, SEEDS is looking at using the model to identify homes in densely populated urban areas that might be susceptible to heatwaves as temperatures hit new records every summer.
“During a heatwave, roofing becomes the most important parameter because maximum amount of the heat gained in the house happens through the roof. Houses with tin sheets often have poor ventilation and are the most vulnerable at this time,” explains Garg.
There are other projects being piloted too. For instance, they are looking if AI could be used to identify vulnerable houses in the Himalayan state of Uttarakhand, which is prone to earthquakes.
“We brought our disaster expertise to the table, but Microsoft’s data science made it possible for us to develop the model from scratch,” says Ranganathan.
“The Sunny Lives AI model that the SEEDS and Gramener teams have created is a leading-edge humanitarian solution that is already saving lives and helping to preserve the livelihoods of people most at risk of natural disasters,” says Kate Behncken, vice president and lead of Microsoft Philanthropies. “The ingenuity and collaboration between these teams is impressive, and I am encouraged by the promise that this solution holds to help better protect people for other severe weather scenarios, such as heat waves. This is exactly the kind of impact we’re looking to support and drive with NGO partners via the AI for Humanitarian Action program.”
Inspired by the results, SEEDS has started building its own technical capabilities after receiving the AI for Humanitarian Action grant from Microsoft.
“At the end of first year, we also started getting consultants to maintain and improve the accuracy of the model. Microsoft has given us access to the source code, so we may reach a stage soon where we will be able to run the model ourselves,” adds Ranganathan.