一位患者在到達急診室不久後出現了明顯的感染跡象。因此,中國醫藥大學附設醫院 (中國附醫) 的醫生請實驗室進行檢測以確定罪魁禍首。
三天後,他們得到了答案:一種抗藥菌。這種細菌已經進化到可以戰勝大多數抗生素,甚至是作為最後手段使用的抗生素,並且可能帶來致命的威脅,困擾著世界各地的醫院。但此時患者即將出院。這是因為中國附醫開發的人工智慧模型已經在一小時內識別出細菌,而標準實驗室測試則需要長達 72 小時。後來醫生開了新的藥物,病人就康復了。
該人工智慧模型是中國附醫「AST.AI 智慧抗藥菌預測系統」 的關鍵支柱,該系統於去年開始被運用於臨床中。從那時開始,中國附醫的患者死亡率下降了 25%,抗生素成本下降了 30%,抗生素使用量下降了 50%,它幫助醫生治療了數千名患者。

中國附醫人工智慧醫學診斷中心主任許凱程說,這只是中國附醫利用 AI 開創醫療新時代的一個例子。中國附醫開發並部署了數百種人工智慧演算法,這些演算法建立在微軟的 Azure 雲平台上,該系統的 12 家醫院每天都在使用這些演算法。 這些團隊定制的人工智慧模型正在幫助醫生診斷癌症和帕金森氏症等疾病。此外,他們也幫助急診室工作人員更快地治療中風和心臟病患者,並減輕了醫生和護士的文書工作負擔。「我們開發每一個人工智慧工具的主要目標都是拯救病人的生命和節省醫生的時間,」許凱程主任說。 「我們的願景是成為未來人工智慧醫院的世界級平台提供商。」
節省時間,拯救生命
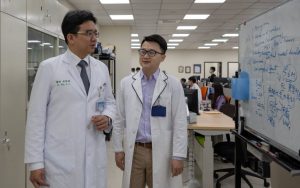
多年來,中國附醫一直在為成為 AI 醫院奠定基礎。 2015年,系統建立了一個大數據中心,在保障患者數據隱私的同時,為AI演算法提供素材。兩年後,中國附醫人工智慧醫學診斷中心成立。現今,中國附醫有 10 家不同的 AI 診所。一個由醫生和 AI 研究人員組成的跨學科團隊每月開會討論潛在的新案例並審查現有研究的進展,目前成功被驗證的模型已經被實施至臨床中。 至今為止,其中 8 個 AI 模型已獲得台灣食品藥品監督管理局的監管批准,另有 8 個已提交審查。以上這些都是建立在 Azure Machine Learning 之上。
「Azure 是我們醫療數位轉型的核心,」中國附醫智慧科技創新中心主任游家鑫博士說。 「該平台讓我們能夠快速開發新的人工智慧工具,將它們實際運用在醫療院所,並改善醫療人員的日常。」
人工智慧模型通常被納入醫生每天使用的熟悉軟體中,有些是透過按一下按鈕來啟動的 : 例如,在病患膝蓋受傷時預約 MRI 的醫生可以點擊標記為「AI」的按鈕來預測半月板撕裂的可能性。即時結果可以避免延誤後續預約並讓患者更快得到治療。
節省時間通常意味著拯救生命。 WHO 世界衛生組織將「病菌抗藥性」列為人類健康的十大威脅,並警告 2050 年時,病菌抗藥性將成為全球最大死因,致死人數超過癌症。 若要鑑定可能致命的抗藥菌,標準的實驗室方法可能需要長達三天的時間。因此中國附醫為此與微軟合作,積極投入相關研究,在 Azure 平台上開發「AST.AI 智慧抗藥菌預測系統」,藉由 Azure Machine Learning 將原本須 2-5 天才能得知的「病菌抗藥性」結果,成功縮短為 1 小時。
許凱程主任認為對於可能會心臟病發作的患者而言,在等待專家審查心電圖(ECG)時,重症監護可能會因此而延遲。中醫大附醫開發了一種演算法,可以分析心電圖和檢測心臟病發作的可能性,並向值班專家發送消息進行確認。
中國附醫急診室工作人員已經使用AI模型兩年了。許凱程主任認為從患者抵達到接受治療所需的時間縮短了一半。這項演算法非常成功,因此他們決定與一家醫療設備製造商合作,將此系統作為預警系統引入救護車。
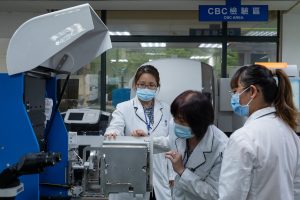
許凱程主任表示,台中市和南投市的消防部門已經使用該系統近一年了。心電圖可以在救護車上拍攝並上傳到雲端,人工智慧模型可以幫助診斷並提醒醫院的團隊為心臟病發作患者做準備,將癥狀發作和開始治療之間的時間從兩個小時縮短到10分鐘。
然而,並非所有的人工智慧模型都如此成功,許凱程主任和游家鑫博士坦承。
中國附醫開發了一種預測敗血症的演算法。敗血症是一種由感染引發的嚴重疾病。許多膿毒症患者表現出器官衰竭的跡象。當人工智慧模型在訓練時,它學會將這些症狀與疾病聯繫起來。但是,並非所有器官衰竭患者都有膿毒症。結果,該模型錯誤地將一些器官衰竭患者標記為患有敗血症。游家鑫博士說,為了解決這個問題,該團隊可以增加額外的標籤來訓練未來的模型,以説明與區分器官衰竭不是由感染引起的情況。
許凱程主任和游家鑫博士指出,醫生總是不得不使用他們擁有的數據做出複雜的決定。人工智慧通過提供更深入與廣泛的數據來幫助支持這些決策,而不是做出留給醫學專家的決策。
基於中國附醫的經驗,人工智慧可以改善醫生提供的幫助。這就是為什麼他們希望使用Azure平臺廣泛共用AI工具的原因。
「我們希望將我們的人工智慧模型部署到世界各地的醫院和診所,使用雲平臺更加高效和具有成本效益」游家鑫博士說。「患者隱私始終是首要關注的問題,我們選擇 Azure 的一個關鍵原因是因為它可以確保敏感健康數據的安全。Azure確實減少了將AI引入醫院的障礙。」
相關:
閱讀關於中國附醫使用AI檢測心臟病發作、發現大腦中阻塞的動脈和抗藥性微生物的相關研究。
原始文章請見 Inside Taiwan’s ‘AI hospital of the future’
The telltale signs of infection appeared soon after the patient arrived in the emergency room. So doctors at China Medical University Hospital (CMUH) ordered a lab test to identify the culprit.
Three days later, they got their answer: a drug-resistant bacteria. This particular bug had evolved to outfox most antibiotics, even ones used as a last resort. It is a notorious and potentially fatal threat that haunts hospitals worldwide.
But by then the patient was about to be discharged. That’s because an AI model developed by CMUH had already identified the bacteria – in one hour, compared with the 72 hours the standard lab test took. Doctors had prescribed new drugs as a result, and the patient recovered.
That AI model is a key pillar of CMUH’s “intelligent antimicrobial system,” which was deployed into clinical practice last year. Since then, CMUH has seen patient mortality fall by 25%, antibiotic costs by 30% and antibiotic use by 50%. It has helped doctors treat thousands of patients much like the one who left the ER in March.
And it is just one example of how CMUH is using AI to create a new era of healthcare, said Dr. Kai-Cheng Hsu, director of CMUH’s Artificial Intelligence Center for Medical Diagnosis.
CMUH has developed and deployed hundreds of AI algorithms, hosted on Microsoft’s Azure cloud platform, that are used every day across the system’s 12 hospitals. The team’s custom-built AI models are helping doctors diagnose diseases like cancer and Parkinson’s. They’re helping ER staff treat stroke and heart attack patients quicker. And they’re helping ease the paperwork load on doctors and nurses.
“The major goals of every AI tool we develop are to save patients’ lives and save doctors’ time,” Hsu said. “Our vision is to be a world-class platform provider for the AI hospital of the future.”
Saving time, saving lives
CMUH has been laying the foundations for that AI hospital for years. In 2015, the system established a center for big data, which could ensure patient data privacy while supplying training fuel for AI algorithms. The AI Center for Medical Diagnosis launched two years later.
Today, 10 different AI clinics operate at CMUH. A cross-disciplinary team of doctors and AI researchers meet monthly to discuss potential new projects and review progress on existing research. Models that are successfully validated and tested are integrated into clinical practice. To date, eight of its AI models have received regulatory approval from Taiwan’s Food and Drug Administration, and eight more have been submitted for review.
All have been developed and deployed using the Azure Machine Learning platform.
“Azure is at the core of our digital healthcare transformation,” said Dr. Jiaxin Yu, director of CMUH’s AI Innovation Center. “The platform lets us quickly develop new AI tools, deploy them into the clinic and improve the daily practice of our physicians.”
The AI models are often incorporated into familiar software doctors use every day. Some are deployed with a literal push of a button. For example, doctors who order an MRI on a knee injury can click a button marked “AI” that predicts the likelihood of a meniscus tear. That instant result can avoid delays for a follow-up appointment and get a patient treated quicker.
Saving time often means saving lives. That is increasingly true when it comes to the rapid identification of deadly, drug-resistant bacteria. The World Health Organization predicts that by 2050, antibiotic resistance will cause more deaths than cancer.
But as the patient with drug-resistant bacteria found, the standard lab method can take up to three days. So CMUH developed a new model. It combined machine learning with a common and much simpler lab technique that identifies molecular fingerprints of different bacterial strains. Data on thousands of clinical samples were used to train the algorithm, which can accurately identify six different types of multi-drug resistant bacteria in one hour, Yu said.
With possible heart attack patients, critical care can be delayed while waiting for a specialist to review an electrocardiogram (ECG), Hsu said. CMUH developed an algorithm that can analyze ECGs, detect the likelihood of a heart attack and send a message to an on-duty specialist for confirmation.
CMUH emergency room staff have used the AI model for two years. It has cut in half the time between when a patient arrives and when they are treated, Hsu said. The algorithm was so successful they decided to partner with a medical device manufacturer to bring it into ambulances as an early warning system.
Fire departments in the cities of Taichung and Nantou have used the system for nearly a year, Hsu said. An ECG can be taken in an ambulance and then uploaded to the cloud, where the AI model can help with a diagnosis and alert medical teams at the hospital to prepare for a heart attack patient. This can cut the time between symptom onset and the start of treatment from two hours down to 10 minutes, he said.
Not all AI models have been as successful, Hsu and Yu said.
CMUH developed an algorithm to predict sepsis, a serious illness triggered by infection. Many patients with sepsis show signs of organ failure. When the AI model was trained, it learned to correlate those symptoms with the illness. But not all patients with organ failure have sepsis. As a result, the model incorrectly flagged some patients with organ failure as having sepsis. To solve the problem, Yu said, the team could train future models with additional labels to help it distinguish cases where organ failure is not caused by infection.
Hsu and Yu noted that doctors have always had to make complex decisions using the data they had. AI is there to help support those decisions by providing deeper insights into data, not to make decisions best left to medical experts.
Based on CMUH’s journey, AI can help improve the care doctors deliver, they said. That is why CMUH hopes to share its AI tools broadly using the Azure platform.
“We want to deploy our AI models to hospitals and clinics around the world, and it is much more efficient and cost-effective to use a cloud platform,” Yu said. “Patient privacy is always a top concern, and a critical reason we chose Azure is because it can ensure the safety of sensitive health data. Azure really reduces the barriers to bringing AI into the hospital.”
Related:
Read CMUH’s peer-reviewed research on using AI to detect heart attacks, spot blocked arteries in the brain and identify drug-resistant microbes.