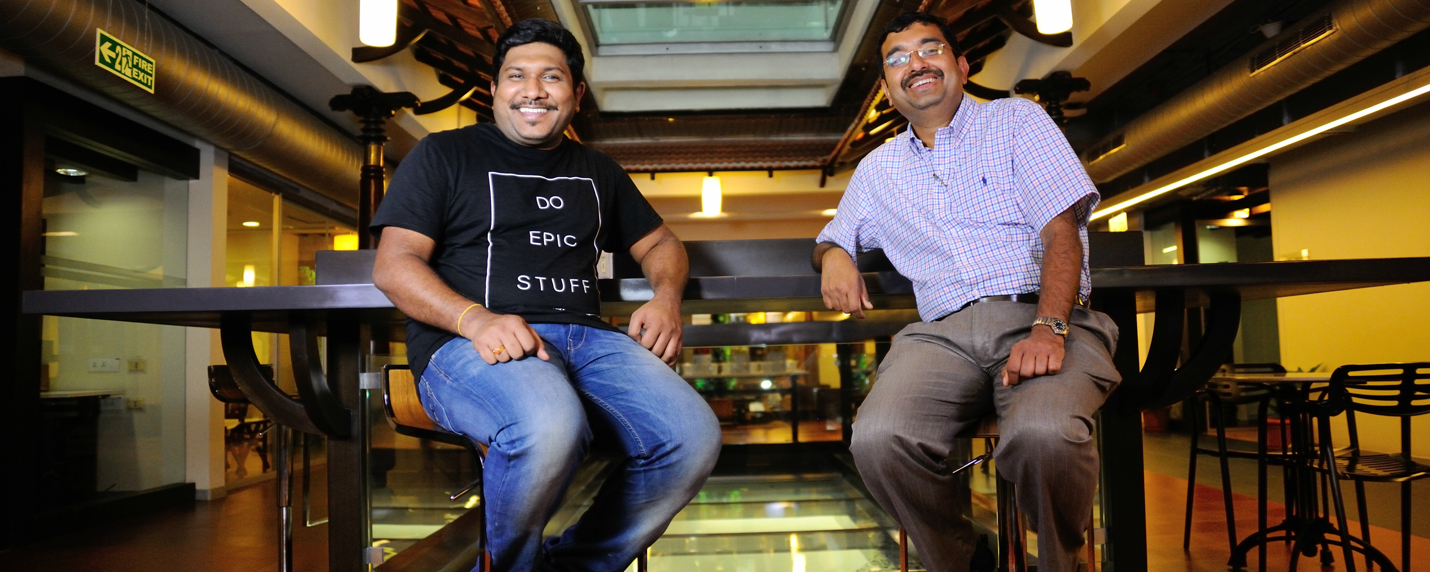
HAMS
Microsoft researchers use visual AI to make India’s roads safer
A nervous student gets into a car. It’s his first time behind the steering wheel. He glances anxiously at his driving instructor on his left. The instructor reassures him and starts on a set of instructions. He urges him to turn on the ignition, slide the gear from neutral to first, and slowly release the clutch while stepping on the throttle. He also reminds him to be ready to brake if needed and to keep an eye on the rear and wing mirrors.
The scenario above is how drivers have been trained at the Institute of Driving and Traffic Research (IDTR)—a joint venture between the Department of Transport of State Governments and car manufacturer Maruti Suzuki India Ltd., India’s largest passenger car manufacturer. Founded in 2000, IDTR’s aim is to make Indian roads safer.
India has one of the highest number of road accidents in the world. In 2016, 17 deaths and 55 road accidents occurred every 60 minutes—one death every four minutes. The main contributing factors are poor road infrastructure, low awareness of road rules and traffic signs, and distracted and inefficient driving.
One way in which IDTR tries to address India’s dismal road safety record is by teaching safe driving, via scientifically engineered training, testing tracks of international standards and through simulators. Some aspects of IDTR’s methodology are also being used by Maruti Driving Schools, an added service offered countrywide by the dealers of Maruti Suzuki India Ltd.
“At IDTR, our primary focus is on providing quality training to the drivers and developing better methods of training. For this, we use technology to a great extent—simulators, and cameras. Recently, we have developed an on-board diagnostic (OBD) device used for in-car automation. This enhances quality of driving training instructions” says Mahesh Rajoria, Director IDTR and head of Driving Schools Division at Maruti Suzuki, The driving schools network comprises of IDTR and the Maruti Driving Schools (MDS). Collectively, IDTR and MDS have trained over three million drivers so far.
However, IDTR now has one more addition, which could change the way trainers teach students to drive cars – an inconspicuous smartphone mounted on the car’s dashboard that records both the driver and the view of the road from the front windshield. After every session, it provides detailed analysis to the instructor, which wasn’t possible earlier. The solution, made by researchers at Microsoft, is called HAMS.
HAMS: Leveraging low-cost tech to tackle road safety
HAMS, which stands for Harnessing AutoMobiles for Safety, is a virtual harness for vehicles that focusses on two factors that are critical to road safety—the state of the driver, and his or her driving relative to other vehicles.
It employs the front and back cameras of dashboard-mounted smartphone, the phone’s GPS and inertial sensors, and an On-Board Diagnostics (OBD-II) scanner, which provides information from the vehicle. Much of this data is processed locally on the smartphone itself, with an Azure-based backend being used for aggregating and visualizing the processed data. The front-camera of the smartphone looks at the driver, the back camera looks out to the front of the vehicle and based on the raw data obtained from the sensors, HAMS detects various events of interest such as driver distraction, fatigue and gaze tracking, as well as vehicle ranging, which determines whether a safe separation distance is being maintained with the vehicles in front.
HAMS monitors driver fatigue by detecting eye closure and yawns from the phone’s front camera. Eye Aspect Ratio (EAR) metric is used to detect eye closure, based on which the PERCLOS metric quantifying the percentage of time the eyes are closed is computed. Yawns are detected using the Mouth Aspect ratio (MAR) metric, which helps detect when the mouth remains open for a continuous period of at least one or two seconds. Gaze tracking, which is done through head pose estimation and eye gaze tracking, enables analyzing mirror scanning behavior, for example, to detect episodes when a driver stares ahead for a prolonged period, thereby failing to maintain awareness of their surroundings.
Vehicle ranging, aimed to prevent tailgating, is determined by delineating a bounding box around the vehicle in front as viewed through the smartphone’s back camera. Based on the size of the bounding box, the distance to the vehicle is estimated.
The success of HAMS lies in its effective architecture that performs edge-based processing of multimodal sensor data using a hybrid approach that combines machine learning with traditional techniques to balance accuracy with efficiency. Such edge-based processing enables raw data to be processed locally on the smartphone, enabling greater efficiency and minimal data usage. It also ensures privacy, since only the detections and no raw images are uploaded to the cloud.
Effective monitoring leading to actionable feedback
“Since we were already working in this direction—that is bringing technology in driving training—we were ready to go along when Microsoft researchers discussed about HAMS,” says Rajoria, recollecting the initial deployment of HAMS with Ashish Mathur from his team.
“HAMS covers parameters in driving instruction that we thought was never possible,” says a jubilant Rajoria. “Take for example, the parameter of maintaining the correct distance between the vehicle you are driving and the vehicle in the front. Now this is a very important parameter as far as the driving instruction goes. HAMS is definitely going to help us with that.”
HAMS is already being used in some cars at IDTR and instructors revisit the footage and analytics after every training session to give feedback to their students in the next session.
The vision behind HAMS
The genesis of the HAMS project goes back to a decade ago, when Principal Researcher Venkat Padmanabhan returned to India after having spent eight-and-a-half years at Microsoft Research in Redmond, USA. “The first thing that hits you, quite literally, is the traffic,” he recalls when asked about how the HAMS project came to be. “Back then, we did a project called Nericell, where we came up with an idea of using smartphones, or what was then considered to be a smartphone, to monitor road and traffic conditions. While we did succeed on many fronts—we did a small-scale deployment and our 2008 paper has garnered well over 1,000 citations and has spawned many efforts—because of the limitations of the hardware, we couldn’t really take it very far.”
All this changed in 2015, when the researchers decided to concentrate the research on road safety, narrowing down on the driver and the driving. The fast developing IoT ecosystem and the advancement of smartphone hardware with faster processors, better cameras and multiple sensors – accelerometer, gyroscope – accelerated the efforts.
“It was the summer of 2016 when we started building and deploying HAMS in over a dozen cabs at Microsoft Research office in Bengaluru—vehicles that were used to shuttle employees back and forth,” Padmanabhan recalls.
But before the successful launch of HAMS, Microsoft researchers had to face challenges on several fronts. First, since HAMS utilized edge processing that would happen directly on the smartphone, the researchers had to figure out how to process information in an intelligent way. Second, the algorithms had to be self-calibrating to work in uncontrolled environments, where, for instance, the mounting of the phone with respect to the driver could vary. The algorithms also required a fair amount of customization to define the various parameters such as vehicle tracking and ranging.
Post-doctoral researcher Akshay Uttama Nambi, who is part of the Microsoft research team that developed HAMS, elaborates on how they overcame the challenges. “Especially with vehicle ranging, where we had to identify the distance between your vehicle and the vehicle in the front, the algorithms had to be efficient enough to track and identify the vehicle in real-time. We developed a hybrid approach where we mix a high computational intensive task with a low computational intensive task. This balances the load on the smartphone.”
“We identified certain features which could be reused across multiple detectors. For example, facial landmarks can be computed once for each image, and then could be used for multiple detectors such as for fatigue, gaze, etc. Thus the heavy-lifting done in extracting the facial landmarks could be used for such diverse tasks as tracking the driver’s blinking rate, detecting whether he is yawning, or whether his gaze was directed in the appropriate direction,” Nambi explains.
Immense possibilities to be explored
The possibility of HAMS goes far beyond it just being used as an aid for driver training. For instance, it could potentially be deployed during the issuance of driving licenses. Presently, just a single practical test along with a theory exam is needed to get a driving licence in India. “If HAMS is deployed, an applicant with a learner’s license can be tested over 100 or 1,000 kilometres, before the licence is granted,” says Padmanabhan.
Another area where HAMS can be put to use is in fleet management, providing stakeholders such as fleet owners or supervisors with visibility in an intelligent way. The fleet can comprise hundreds or thousands of cabs, buses, or trucks, being overseen by a supervisor.
Parents could also possibly use HAMS to monitor the driving of their teenage kids, who might be new drivers.
“Different markets can have dramatically different needs, and this is evident in the innovations in the automotive industry. While self-driving cars are being actively worked on in the West, there is a huge need in India and emerging markets to use AI in existing human-driven cars to help the driver drive safely,” says Sriram Rajamani, Managing Director, Microsoft Research India.
“There is also a huge need to improve safety of fleets such as truck fleets, bus fleets and car fleets. HAMS is an extremely interesting project because it deals with existing vehicles, and existing fleets, and explores improving safety while being frugal in terms of costs,” he adds.