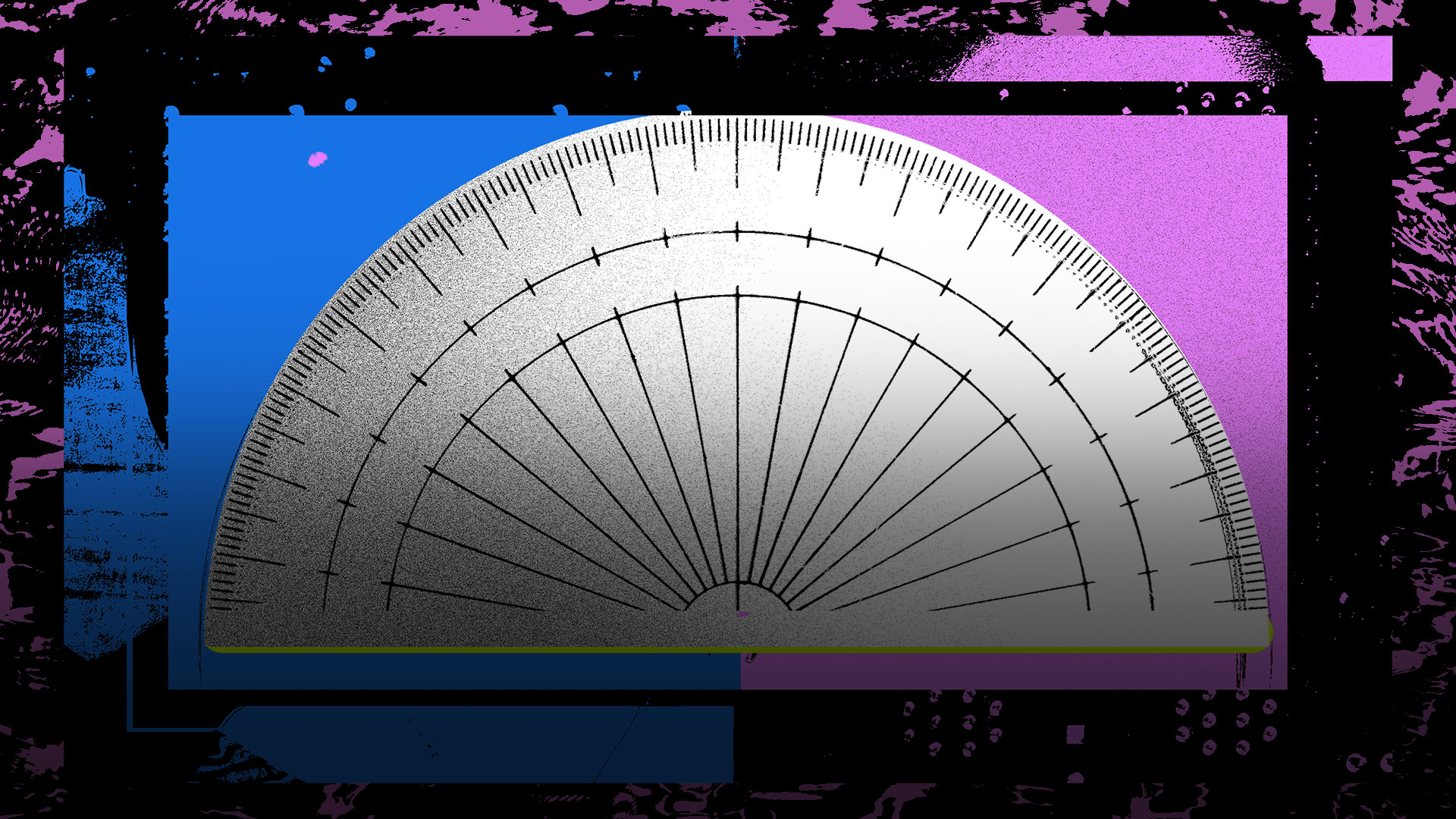
Measurement is the key to helping keep AI on track
When Hanna Wallach first started testing machine learning models, the tasks were well-defined and easy to evaluate. Did the model correctly identify the cats in an image? Did it accurately predict the ratings different viewers gave to a movie? Did it transcribe the exact words someone just spoke?
But this work of evaluating a model’s performance has been transformed by the creation of generative AI, such as large language models (LLMs) that interact with people. So Wallach’s focus as a researcher at Microsoft has shifted to measuring AI responses for potential risks that aren’t easy to quantify — “fuzzy human concepts,” she says, such as fairness or psychological safety.
This new approach to measurement, or defining and assessing risks in AI and ensuring solutions are effective, looks at both social and technical elements of how the generative technology interacts with people. That makes it far more complex but also critical for helping to keep AI safe for everyone.
This post is part of Microsoft’s Building AI Responsibly series, which explores top concerns with deploying AI and how the company is addressing them with its responsible AI practices and tools.
“A lot of what my team does is figuring out how these ideas from the social sciences can be used in the context of responsible AI,” Wallach says. “It’s not possible to understand the technical aspects of AI without understanding the social aspects, and vice versa.”
Her team of applied scientists in Microsoft Research analyzes risks that are uncovered by customer feedback, researchers, Microsoft’s product and policy teams, and the company’s AI Red Team — a group of technologists and other experts who poke and prod AI systems to see where things might go wrong.
When potential issues emerge — with unfairness, for example, such as an AI system showing only women in the kitchen or only men as CEOs — Wallach’s team and others around the company step in to understand and define the context and extent of those risks and all the different ways they might show up in various interactions with the system.
Once other teams develop fixes for any risks users might encounter, her group measures the system’s responses again to make sure those adjustments are effective.
She and her colleagues grapple with nebulous concepts, such as what it means for AI to stereotype or demean particular groups of people. Their approach adapts frameworks from linguistics and the social sciences to pin down concrete definitions while respecting any contested meanings — a process known as “systematization.” Once they’ve defined, or systematized, a risk, they start measuring it using annotation techniques, or methods used to label system responses, in simulated and real-world interactions. Then they score those responses to see if the AI system performed acceptably or not.
The team’s work helps with engineering decisions, giving granular information to Microsoft technologists as they develop mitigations. It also supports the company’s internal policy decisions, with the measurements helping leaders decide if and when a system is ready for deployment.
Since generative AI systems deal with text, images and other modalities that represent society and the world around us, Wallach’s team was formed with a unique mix of expertise. Her group includes applied scientists from computer science and linguistics backgrounds who study how different types of risks can manifest. They partner with researchers, domain experts, policy advisors, engineers and others to include as many perspectives and backgrounds as possible.
As AI systems become more prevalent, it’s increasingly important that they represent and treat marginalized groups fairly. So last year, for example, the group worked with Microsoft’s chief accessibility officer’s team to understand fairness-related risks affecting people with disabilities. They started by diving deep into what it means to represent people with disabilities fairly and identifying how AI system responses can reflect ableism. The group also engaged with community leaders to gain insight into the experiences people with disabilities have when interacting with AI.
Turning those findings into a clearly systematized concept helps with developing methods to measure the risks, revise systems as needed and then monitor the technology to ensure a better experience for people with disabilities.
One of the new methodological tools Wallach’s team has helped develop, Azure AI Studio safety evaluations, uses generative AI itself — a breakthrough that can continuously measure and monitor increasingly complex and widespread systems, says Sarah Bird, Microsoft’s chief product officer of responsible AI.
Once the tool is given the right inputs and training in how to label an AI system’s outputs, it roleplays — for example, as someone trying to elicit inappropriate sexual content. It then rates the system’s responses, based on guidelines that reflect the carefully systematized risk. The resulting scores are then aggregated using metrics to assess the extent of the risk. Groups of experts regularly audit the testing to make sure it’s accurate and in alignment with humans’ ratings, Bird says.
“Getting the AI system to behave like the experts, that’s something that takes a lot of work and innovation and is really challenging and fun to develop” as Microsoft invests in the evolving field of evaluation science, she says.
Microsoft customers can use the tool, too, to measure how their chatbots or other AI systems are performing against their specific safety goals.
“Evaluation is the robust thing that helps us understand how an AI system is behaving at scale,” Bird says. “How will we know if our mitigations and solutions are effective unless we measure?
“This is the most important thing in responsible AI right now.”
Read our first two posts in the series on AI hallucinations and red teaming.
Learn more about Microsoft’s Responsible AI work.
Lead illustration by Makeshift Studios / Rocio Galarza. Story published on September 9, 2024.