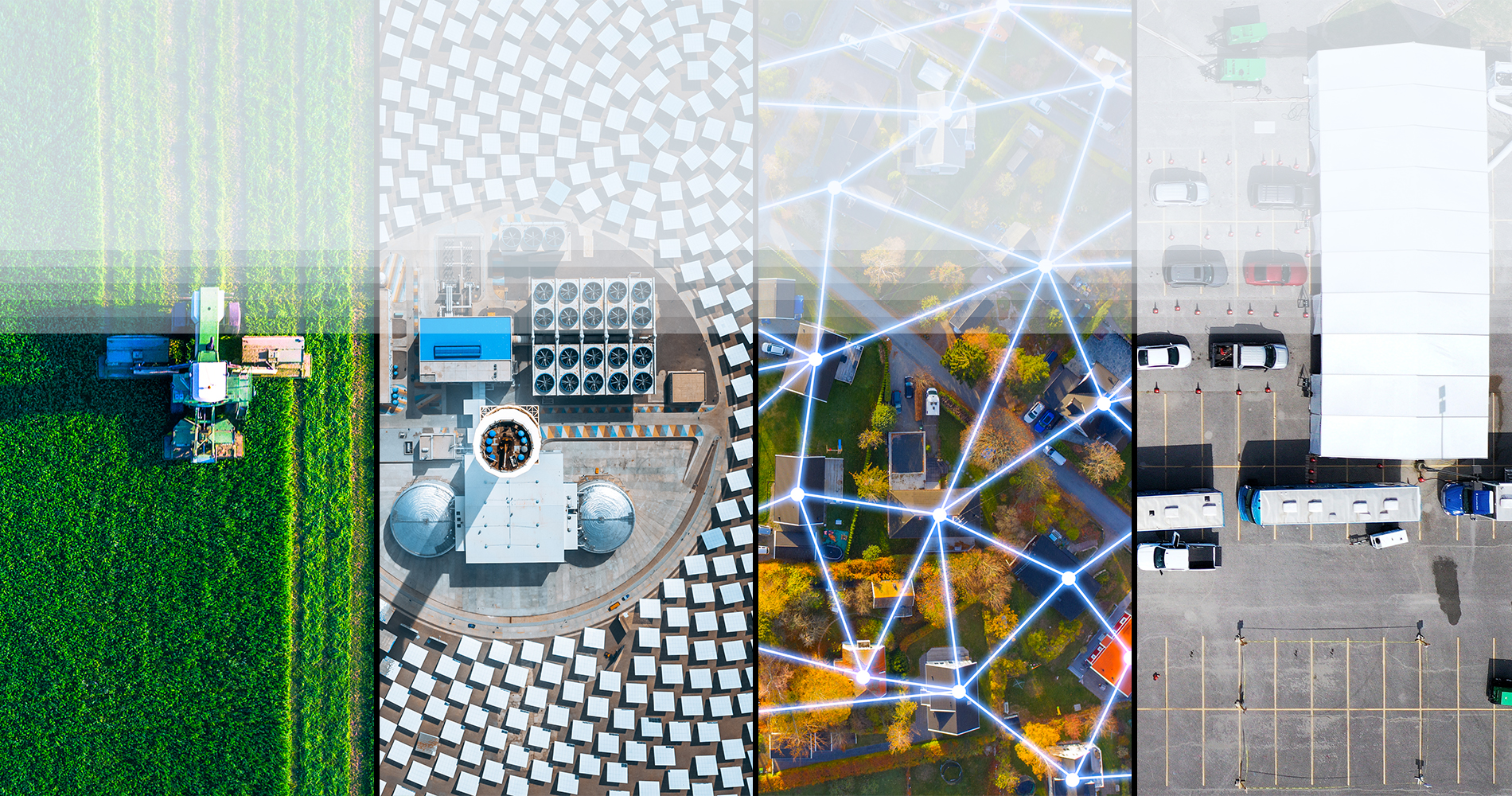
Open Data for Social Impact Framework
Introduction Making the most of open data and data collaboration
A note from Burton Davis
At Microsoft, we believe that data is critical to addressing the important social problems our world faces today. The global pandemic has shown us the important role of data in understanding, assessing, and taking action to solve the challenges created by COVID-19. However, nearly all organizations, large and small, still struggle to make data relevant to their work. Despite the value data provides, many organizations fail to harness its power to improve outcomes.
Part of this struggle stems from the “data divide” – the gap that exists between countries and organizations that have effective access to data to help them innovate and solve problems and those that do not. To close this divide, Microsoft launched the Open Data Campaign in 2020 to help realize the promise of more open data and data collaborations that drive innovation.
One of the key lessons we’ve learned from the Campaign and the work we’ve been doing with our partners, the Open Data Institute and The GovLab, is that the ability to access and use data to improve outcomes involves much more than technological tools and the data itself. It is also important to be able to leverage and share the experiences and practices that promote effective data collaboration and decision-making. This is especially true when it comes to working with governments, multi-lateral organizations, nonprofits, research institutions, and others who seek to open and reuse data to address important social issues, particularly those faced by developing countries.
Put another way, just having access to data and technology does not magically create value and improve outcomes. Making the most of open data and data collaboration requires thinking about how an organization’s leadership can commit to making data useful towards its mission, defining the questions it wants to answer with data, identifying the skills its team needs to use data, and determining how best to develop and establish trust among collaborators and communities served to derive more insight and benefit from data.
The Open Data for Social Impact Framework is a tool leaders can use to put data to work to solve the challenges most important to them. Recognizing that not all data can be made publicly accessible, we see the tremendous benefits that can come from advancing more open data, whether that takes shape as trusted data collaborations or truly open and public data. We use the phrase ‘social impact’ to mean a positive change towards addressing a societal problem, such as reducing carbon emissions, closing the broadband gap, building skills for jobs, and advancing accessibility and inclusion.
We believe in the limitless opportunities that opening, sharing, and collaborating around data can create to draw out new insights, make better decisions, and improve efficiencies when tackling some of the world’s most pressing challenges.
Burton Davis
Vice President and Deputy General Counsel, Intellectual Property Group at Microsoft
This site is not intended to provide legal guidance and should not be relied on as such. The framework provides resources to help organizations advance open data and engage in data collaborations for social good. Sharing data is not risk-free. It is recommended to obtain independent legal advice. This site includes links to third party resources. Microsoft is not responsible for any loss, injury, or damage arising out of the use of the links or reliance on materials made available by third parties.
Quick Start: Your Roadmap to Open Data A simple roadmap to getting started
Explore the roadmap
Organizations interested in using open data may understand its benefits and how it could apply to specific projects but how to take that first step may be unclear. The following is a simple roadmap that you can follow to start using more open data to address challenges in your organization, community, and the greater world. Where indicated, click to learn more and jump to the corresponding sections in this framework.
1. Determine if you have the organizational infrastructure in place.
For many organizations, adopting an open data approach is a cultural shift. Do you have buy-in from your stakeholders? Have you assessed any investments needed to put data to work? Is trust established across stakeholders? Make sure you have these answers before taking the next step.
Learn more in Leadership: Are you ready to put data to work to improve social outcomes?
2. Understand the questions you want to answer with data.
Your data strategy begins by identifying the questions you are looking to answer. Once you identify these questions, you can determine which datasets are needed and whether you have access to them.
Learn more in Opportunity: What are the questions you want to answer with data?
3. Assemble the necessary talent.
You need the right strategy and the right datasets. But you also need the right people to provide analysis and insight over the course of your project. Make sure you evaluate the data skills required and that all your team members understand that collaboration is at the heart of the project.
Learn more in Talent: Do you have the talent needed for data analysis?
4. Build trust in the community.
Create a good governance framework to ensure that both data opportunities and data risks are addressed. Make sure your governance policy ensures transparency among stakeholders, inclusivity among community members, and fosters enablement, so they understand why gathering and using open data is in their best interest.
Learn more in Community Governance: Have you built trust in your community around the use of data?
5. Make sure you have the right data resources.
Analytics and data visualization tools are essential if you are working across a broad range of datasets and a deep volume of data. Similarly, ensuring any privacy and security needs are accounted for is essential to responsible open data and data sharing collaborations.
Learn more in Technology and Data: Which solutions and resources do you need to enable and enhance your impact?
Background and Context Challenges of Open Data Explained
Why is access to data so important?
Artificial intelligence, or AI, is the backbone of the digital transformation the world is currently experiencing. Industrial operations, business processes, customer management, and more are being transformed by machine learning that is creating the opportunity for greater experimentation, efficiency, and speed. Streamlining the collection, storage, and management of large amounts of data creates more reliable insights which organizations can use in their decision-making.
Large and diverse datasets can help strengthen those insights or add to them. Open data, which is data that is published for anyone to access, use, and share without restriction, can help users solve problems at greater speed and with greater authority, which can lead to faster breakthroughs and, because it can also be predictive, can generate forecasts with greater accuracy. For these reasons, the value that open data offers in healthcare, science, education, the environment, and more is immeasurable for helping solve society’s greatest challenges.
But by opening data up, won’t it lose value?
In most cases, data by itself is not where the value lies, the value comes from what you do with that data. In this context, it’s also important to note a few economic aspects of data. Data is non-rivalrous – it can be used again and again by many without depleting value, and it can be used to achieve network effects – for example, more data can create better AI, which can attract more usage and generate more data.
Opening data up or making data more accessible has the potential to generate more value than keeping it siloed and it can unlock enormous public value. Users who combine the data with other datasets or work with it in a new context may uncover new insights that were not apparent in its original use. As the Open Data Policy Lab cites in its 9Rs Framework, more open data can also enable reproducibility, improving confidence in results by allowing others to conduct identical or related work.
The key is not simply transferring data to other users but working with them to understand how it is being used and how the benefits of those uses can be shared back with the community. Its value comes from how it is used to facilitate new meanings and solutions. Opening up data can help to avoid the missed uses – and missed value of data if it is kept closed. This is the collaborative spirit of open data. Its value is endless.
For more on the value of data, visit the Open Data Institute’s The Value of Data report.
What about privacy concerns associated with opening data up?
A concern with opening up data may be the risk of disclosing sensitive data. Safeguarding individual privacy and protecting confidential or commercially sensitive information may be required by law or governed by contract. In addition, organizations must also consider the reputational, ethical, and commercial risks for sharing sensitive data.
To protect stakeholders in the data sharing ecosystem, and to engender trust in data sharing, it is important to protect sensitive data through the appropriate legal, technical, and organizational means. But this requirement should not deter organizations from pursuing an effective data strategy. Rather, the level of protection can be achieved by implementing suitable governance frameworks for responsible data sharing.
For example, privacy enhancing tools can be used to help keep personal information private. Technologies and techniques such as differential privacy, homomorphic encryption, confidential computing, anonymization, and de-identification can be leveraged to safeguard individual privacy while enhancing access to data by organizations, researchers, and civil society. While these technologies may not be appropriate for all settings, they can be useful in certain contexts.
For more on the enabling conditions and disabling factors that often determine the impact of open data initiatives, visit The GovLab’s Periodic Table of Open Data’s Impact Factors.
The Benefits of Open Data for Your Organization
It Creates More Informed Decision-Making
Open data can give stakeholders new knowledge that helps them make more informed and objective decisions. The value of additional datasets from different sources can help users gain greater clarity around issues and unlock new insights. As the dictate goes, “you don’t know what you don’t know.” Open data presents an opportunity to uncover new possibilities users had previously never considered.
Driving this process is the nature of open data itself. For example, data can be analyzed in numerous ways to reveal patterns to gain a multi-faceted view of the problem the user is trying to solve. Those findings can be shared with the broader public for crowdsourcing to enhance the findings or create new ones not yet discovered. Alongside broader sharing and awareness, open data can encourage others to reciprocate and can yield contributions that benefit everyone.
As an example, The Nature Conservancy (TNC)-India and Microsoft are using existing open satellite imagery to create a new open dataset on solar farms in India. This data will help to identify factors driving land suitability for solar projects and, ultimately, help public agencies better plan for solar energy development.
Decision-making, therefore, does not have to be strictly based on datasets that reside within any one organization. Open data enables access to data that is published by others, and it can create the opportunity for further inputs to your organization’s data, further informing the end result and decision-making.
Which Creates New Opportunities for Discovery
As suggested above, open data helps users identify and address different problems that may not have been initially considered. Open data also helps organizations identify connections with other datasets. The Purdue Food and Agricultural Vulnerability Index drew on vastly different open datasets to generate new insights into the impact of COVID-19 on farm production and the health of farmers and farmworkers.
With access to more data, insights can be gained faster. This gives users freedom to experiment with new ideas, to see correlations not known before, or to prolong the discovery phase. This continual unfolding of the data allows new possibilities in ways that can be more efficient than existed previously.
As a Result, Innovation Can Be Expedited
Breakthroughs in science using open data have already shown us that it represents an important model for researchers, one that promotes sharing protocols, the reporting and disseminating of results, sharing code, and more. The very nature of any kind of research is indeed contingent on making sure data is searchable, accessible, and reusable to drive third-party scrutiny.
The promise of open data is that it opens those doors and expedites research and innovation for public gain. For example, making certain health data shared or public helped accelerate the development of medical treatments like the vaccines produced to combat the COVID-19 virus. Lessons learned from that experience have motivated the U.S. to allocate billions of dollars to support more timely research. The National Institutes of Health has supplied funds of almost $4.9 billion to date to support COVID-19 research projects. Incorporating open data principles into these programs can help accelerate research, which will benefit the current pandemic as well as crises that lay ahead.
The Value of Open Data – By the Numbers
- In a 2013 report by McKinsey Global Institute, the open data market, valued at $3 trillion a year, is centered on the value of combining open government data with shared data held by businesses.
- In 2014, Lateral Economics estimated that the potential value of open data to the G20 would be around $2.6 trillion a year, contributing to aggregate G20 countries’ cumulative gross domestic product (GDP) of around 1.1% from 2014–2019, or 55% of the G20’s 2% additional growth target.
- In 2020, the European Data Portal estimated that the value of open data for the EU28+ was €184.45 billion in 2019, and forecast it to reach between €199.51 and €334.20 billion by 2025. The report also looked at employment figures, with 1.09 million open data employees in 2019 and 1.12 to 1.97 million open data employees forecast by 2025.
- Transport for London has reported that use of its open data has allowed private sector companies to contribute between £12 million and £15 million per year to the London economy.
For additional insights and case studies on why businesses are embracing the sharing of data, visit the Open Data Institute’s “Seven reasons why businesses should be sharing data”.
For more on the business case for data collaboration and re-using data in the public interest, view the Open Data Policy Lab’s 9Rs Framework.
The Open Data for Social Impact Framework A tool leaders can use
About the framework
The Open Data for Social Impact Framework is a tool leaders can use to put data to work to solve important societal issues, such as reducing carbon emissions, closing the broadband gap, building skills for jobs, and advancing accessibility and inclusion. The following framework is designed to guide organizational leaders across the data ecosystem – governments, nonprofits, and multi-lateral organizations – to insights and solutions they can use to help address important social issues.
This site identifies five topic areas that organizations should consider when seeking to use data to improve social outcomes: leadership, opportunity, skills, community governance, and technology and data. It proposes questions to ask and offers resources that can help answer them. These concepts are brought to life through examples from real-world open data projects. There is also a roadmap to open data that organizational leaders can use to get started.
This framework can serve as a tool to help lay the groundwork for open data and data collaboration. However, there are many other excellent resources to draw upon that can help those wanting to use data for social impact, some of which we identify throughout this site.
The framework promotes a culture of open data and data collaboration by guiding organizational leaders through the following questions:
1. Leadership: Are you ready to put data to work to improve social outcomes?
2. Opportunity: What are the questions you want to answer with data?
3. Skills: Do you have the talent needed for data analysis?
4. Community Governance: Have you built trust in your community around the use of data?
5. Technology and Data: What solutions and resources do you need to measure, enable, and enhance your impact?
1. Leadership Leadership: Are you ready to put data to work to improve social outcomes?
Adopting an open approach is a cultural shift
Leaders of organizations may face a range of concerns or resistance when putting data to work to solve tough challenges. In some of these cases, the talent – with roles ranging from data scientists and data analysts to program managers and researchers – is not in-house to ingest and analyze data. In other cases, the long lead time required to develop a data sharing governance structure with other organizations may result in the collaboration being dropped before it pays dividends.
Ultimately, for most organizations, adopting an open data approach is a cultural shift.
Here, it is important to recognize that organizations fall on a spectrum of data maturity – from an early commitment to using data for innovation to a culture where data innovation is embedded at every level. Regardless of where an organization is along this spectrum, an open data approach requires a leader who is committed to putting an organization’s data to work. This commitment can take a variety of forms, such as:
- Advocating and publicly talking about the importance of sharing data.
- Drawing insights from data.
- Encouraging collaboration and community engagement.
- Building relationships with key beneficiaries and potential data users.
- Setting a framework for the responsible use of data.
These are all actions that help build a trustworthy approach to data sharing that can instill trust among stakeholders.
Steps to consider
Consider the following steps to better position your organization to innovatively use data to solve priority issues:
- Which investments are needed to put data to work?
- Which incentives are needed to put data to work?
- How do you build trust internally and externally? Who are your partners/stakeholders?
- How can you build momentum within your organization to consider innovation with data as a long-term priority, and not a short-term project?
Strong leadership is required
Not surprisingly, organizations that prioritize data as a vital resource require strong leadership. According to a survey published in July 2021 by Data Orchard, 63% of respondents say the leadership in their organization is not convinced about the value of data. Only a third say their leadership is engaged and supportive, ask the right questions of the data, and are active in harnessing its value. The promise of leveraging data creates a significant opportunity for leaders to improve their capabilities of using data to the benefit of their organizations.
The GovLab at NYU hosts a Data Stewards Academy, which includes a self-directed learning program. The course is designed for individuals who serve as data stewards in varying capacities around the world – a function that seeks to answer the questions listed above in a manner that enables systemic, sustainable, and responsible data collaboration.
Profile: How the World Health Organization’s leadership transformed its culture to be data-driven
Cultural, strategic, and operational shifts are all necessary to implement open data and data sharing initiatives. To realize the potential of these benefits – including accountability for results, trust, transparency, and security – leadership must first address internal barriers and other types of organizational resistance. This may include challenging the status quo, implementing large-scale reforms, or taking on a new set of risks – for all these changes, and especially in a large organization, strong leadership and commitment is vital.
When transforming an organization’s culture to be more data-driven, the World Health Organization (WHO) continually demonstrates this necessity. With more than 8,000 employees globally and accountability to its 194 Member States across six regions, the implementation of a digital transformation initiative could only be accomplished through strong leadership and action at every level.
As a multilateral organization, WHO has a unique status as a science- and evidence-based entity that sets globally applicable norms and standards with the mission to promote health, keep the world safe, and serve the vulnerable. When Dr. Tedros Adhanom Ghebreyesus was appointed WHO Director-General in 2017, he recognized that data was a critical component of achieving this mission and meeting the ‘triple billion’ targets of one billion more people enjoying better health and well-being, one billion more people with access to affordable universal health coverage, and one billion more people better protected from health emergencies.
In 2019, Dr. Tedros demonstrated his commitment to transform WHO into a modern, data-driven organization by publicly announcing his vision and establishing a new Division for Data, Analytics and Delivery for Impact (DDI). This division was formed to urgently address data gaps, reduce data fragmentation, and increase efficiencies in WHO’s end-to-end data processes. Particular emphasis was placed on the consolidation of health data and assets for external and internal users as well as the use of modern technologies, including security for private and sensitive data, transparent analytics, and powerful visualization methods.
From the outset, WHO leadership set out to build trust and continuity with both its internal and external stakeholders by promoting a strategic and coherent approach to data governance. Internally, a Data Governance Committee, comprised of senior leadership, was formed to set the corporate direction for data strategy and policy. A Data Hub and Spoke Collaborative was also created to facilitate the implementation of data governance policies across WHO, with all relevant programs and every region represented. Senior leadership were tasked with regularly supporting the meetings of the collaborative to provide guidance, thus encouraging progress and giving much needed advocacy to institutionalize a new data governance mechanism.
Externally, WHO sought outside counsel and partnered with non-UN organizations, including the private sector, to advance its data and analytical capabilities. In June and September 2021, they convened two Health Data Governance Summits to bring together WHO, Member States, partners, and the general public to review best practices and underscore the need for health data as a global public good.
Additionally, in partnership with Microsoft and Avanade and others, WHO leadership made a long-term investment to develop and maintain the technical systems needed to drive decisions through timely, reliable, and actionable data. This investment resulted in the development of the World Health Data Hub (WHDH). The WHDH is the world’s first comprehensive, end-to-end solution for global health and aims to streamline processes and ensure data is accessible, findable, and usable for all stakeholders.
Throughout this transformation process, WHO leadership has demonstrated this commitment by speaking publicly, being visible and engaged both internally and externally, and making long-term investments in the practical tools (such as the WHDH) and the behavior change (such as an updated values charter) needed for implementation. This large-scale cultural shift to a data-driven organization would be impossible without leadership commitment, and WHO can serve as an example for other organizations aiming to make a similar shift.
2. Opportunity Opportunity: What are the questions you want to answer with data?
Understanding “why” is essential to initiate a project
Understanding why you want to solve a problem seems like a simple enough task. In fact, asking “why” may seem so straightforward that this step can often be overlooked. However, asking “why” is essential to initiate a project and to also maintain a sustainable solution. It is required throughout the lifecycle of the innovation, from building momentum within an organization, informing stakeholder engagement, driving progression in the project, deriving the correct approach to governance, ensuring the data is fit for purpose, to implementing a technical solution.
Identify the questions you need to answer to solve your problem
Identifying the questions that you want to answer is a crucial first step. Once the questions are identified, you can start to think about the solutions needed to address them and help solve the problem.
Examples of problems open data may help solve include:
- My organization has data that could contribute to improved mobility planning in my region. How can I share this data in a meaningful and responsible way?
- My organization has published open data on racial inequities. How do we encourage use of this data?
- What are the most cost-effective and equitable interventions to improve air quality in each region, particularly for the pollution sources affecting low- and middle-income countries?
For this last example and other pressing, high-impact questions that could be addressed if relevant datasets were leveraged in a responsible manner, visit The GovLab’s The 100 Questions Initiative.
Understand how data can help you answer those questions
Now that you have identified the questions, the next step is to understand where your organization is on a path towards getting those answers. To do this, it can be helpful to map the current data ecosystem. Mapping the data ecosystem can be used to explore new sources of data, exploit existing data flows, identify where changes are needed, and identify other stakeholders that are also working to solve the same or a similar problem.
One way to get started is to map data actors in your data ecosystem and how value is exchanged across it. For example, the value may come in the form of data, but it also may be the exchange of feedback or knowledge.
For a Data Ecosystem Mapping exercise, visit the Open Data Institute’s Data Ecosystem Mapping: Tool and Guidance.
Determine the value for your stakeholders
Providing access to data can be a critical part of the exercise, but it’s not the only element to consider. It’s also important to consider more broadly how all stakeholders across your data ecosystem will realize value.
Internally, senior leaders will need to gain buy-in from stakeholders and build momentum within the organization to tackle the challenge. External stakeholders will need to understand the alignment with their interests and have incentive to engage. In other words, they’ll need the answer to the question, “what’s in it for me?”
Taking stakeholder interests into account will ultimately help build trust. This can include involving your stakeholders as part of the discussion on value exchanges, such as by attending a group data ecosystem mapping session.
Identify which datasets will help
Trying to assess the data landscape is a daunting task. Once you have clearly defined the questions you want to answer, the step of identifying datasets becomes a more manageable task. Assess the data you have and identify which open and shared data is needed to help solve the problem. You can accomplish this by:
- Using a checklist to identify the data you have and what you can do with it, for example:
- What does the dataset comprise of?
- What aspects do you need to protect and how sensitive is the data?
- Where is the data sourced? Understanding the pedigree of the data is a key component to foster trustworthy use of data. The “pedigree” refers to the quality of the dataset and is based on several factors, including the provenance of the dataset (including metrics of estimated reliability, confidence, and risk).
- Where is the data stored?
- What purpose will it be used for?
- Are there any restrictions on access or use?
- Identifying gaps in data and identifying partners that can contribute to the project or resources to obtain open data.
- Leveraging openly available datasets, such as those made available under open data terms on Azure Open Datasets, GitHub, and Microsoft Research Open Data.
- Preparing your internal datasets for external sharing. For data that can be made available as open, it is recommended to utilize the Community Data License Agreement – Permissive, Version 2.0 (CDLA-Permissive-2.0) or another open data license to share your data. Terms help users to understand the conditions and restrictions governing the use of the data. Attaching appropriate terms identifies the data as open, creates clarity around re-use, and fosters innovation. Nonprofits can access the Microsoft Nonprofit Innovation Hub, which includes a lightweight legal template for establishing a data collaboration.
- Partnering with other organizations or stakeholders that are trying to solve the same problem.
Profile: Caring for Equality through Data Collaboration
In early 2021, the Open Data Institute and Microsoft launched a Peer Learning Network with the aim to help organizations collaborating around data more effectively tackle the challenges they face. This included exploring issues associated with trust and trustworthiness between participants and other stakeholders.
In the first workshop, participants were introduced to the Data Ecosystem Mapping tool to explore the flows of data and value in their ecosystems. This included overcoming barriers to sharing data by developing a trustworthy ecosystem map to understand where trust – or the lack thereof – impacts the value created by data flows.
One of the Peer Learning Network collaboration projects, Caring for Equality, a collaboration of the Government of Buenos Aires City in Argentina, the Center for Global Development, and the Open Data Charter, aimed to address inequality gaps with respect to care-related tasks that constrain women’s economic autonomy. Using data from multiple private and public sources, teams created a “Caring Indicator System” that would address the situation in Buenos Aires and provide information to improve policymaking and accountability to the city’s citizens.
The collaboration used the Data Ecosystem Mapping exercise to identify the data providers and sources, how the data could be accessed and leveraged, and how to consider the trust challenges for sharing data with and within the government. This exercise led to important decision points to help lead the initiative forward with a common understanding of the value of the Care System being built.
More information about Caring for Equality can be found here.
Profile: How London Used Open Data to Better Understand Charging Capacity for Electric Cars
When London announced its plan to be a zero-carbon city by 2030, there was a need to consider greener methods of transport throughout the city and surrounding suburbs. Given that switching from using petrol- and diesel-driven vehicles to electric vehicles can help reduce carbon emissions, the city needed to better understand opportunities to create a better infrastructure for electric charging. That entailed enabling developers and charging point operators to work together to create richer datasets to understand the demand of electric vehicle (EV) owners.
A data sharing pilot was created to understand how the EV charging infrastructure within London could be improved. The public-private data sharing program developed insights to determine potential locations for EV charging stations. The data sharing pilot demonstrated the potential of data sharing, data collaboration, and open data to help develop London’s EV charging infrastructure and ultimately support London’s goal to be carbon neutral by 2030.
Datasets including traffic behavior data, data on charging capacity, and data from the land registry helped identify more than 2,000 public parcels of public land in the city that should be considered further as candidate locations for EV charging points.
The transparency of the data enables the city to demonstrate the basis for analysis, thereby helping to generate trust among skeptics and motivation among investors in EV charging infrastructure. Third parties can scrutinize the analysis to consider whether the conclusions reached are reliable and accurate. Overall, the use of open data in the project showed that, through data sharing, overcoming infrastructure barriers was possible for such a large-scale endeavor.
More information about the EV Charging Infrastructure pilot can be found here.
3. Talent Talent: Do you have the talent needed for data analysis?
Having the skills necessary to work with data is vital for any organization
Just as access to data is critical for organizations to problem-solve and innovate, having the skills necessary to work with that data is vital for any organization. Yet, according to figures from LinkedIn, around half of all people with technical AI skills work in the technology sector and are often in short supply in other organizations and sectors.
When you seek to put data to work for your organization, it is critical to make sure your organization has the talent necessary to architect and execute on a plan to achieve the insights and answers you want to achieve. This does not mean you have to employ teams of computer scientists. To the contrary, a variety of professions and skillsets work with data in different forms, including data analysts, data scientists, software engineers, and researchers. The talent you need will be driven by the data skills needed for your initiative.
Evaluating the data skills needed
A checklist of questions for your organization to use for that evaluation may include:
- What critical data skills are needed to address the challenge identified? For example, technical skills could include managing systems and infrastructure for data processing, implementing data pipelines and analytics, and visualizing or reporting on data. Non-technical skills needed could include consultative requirements gathering, stakeholder management, and program management.
- What data skills do you have within your organization today?
- Where do you have a critical skills gap?
- Can you partner with another organization to fill this gap, or will you need the talent within your own organization?
- Do you need to hire new talent? Is there an opportunity to upskill current talent?
- Do you offer training programs today to help advance data skills? What training programs may be needed?
Detailed descriptions of key technical and business roles for an interdisciplinary team can be found in “The AI playbook,” downloadable here.
For additional resources, refer to the Open Data Institute’s Data Skills Framework.
Profile: The Benefits of Crowdsourcing Using Open Data
Crowdsourcing using open data helps organizations solve difficult problems because it can lead to unexpected solutions, faster problem-solving, and a reduced user burden. Crowdsourcing works by using volunteer (or paid) data collection agents who may or may not have direct ties to the organization conducting the research. Their help ultimately can reduce costs and time by augmenting current skills and systems. Advancements in mobile technology have helped drive the popularity of crowdsourcing because more people now have greater access to data and a breadth of communities around the world.
The benefits of crowdsourcing include:
- Diverse data. Because contributors may reflect a range of users from all over the world, their input is likely to reflect the diversity needed to create the most reliable results.
- Reduced costs. By outsourcing data collection, organizations may be able to reduce costs and fewer resources may be needed to source, clean, and structure datasets inside the organization.
- Greater trust. The name “crowdsourcing” itself implies that data is sourced from outside any one organization and taps into a wide range of contributors. Because of this, the process can gain credibility. This enhanced trust may generate greater participation among the public to help in the research.
A good example of crowdsourcing in the medical field is Folding@home, an organization and online platform that uses crowdsourcing to accelerate simulations, like those that make up the coronavirus responsible for COVID-19, and to develop new therapies.
Through a partnership with Microsoft AI for Health, Dr. Greg Bowman, a molecular biophysicist at Washington University School of Medicine in St. Louis, solicited volunteers from around the world to use their personal computing power to run protein simulations and send the data generated back to its servers. The collective passion to solve a global pandemic resulted in the number of devices running Folding@home to grow from around 10,000 to 1 million in just two months. Bowman sees the crowdsourcing method of using open data as a model to combat both existing and future diseases.
“We can take a problem that would have taken 500 years to complete on a single desktop and solve it in a matter of six months,” he said.
Learn more about Folding@home and Dr. Greg Bowman.
More information about Microsoft’s AI for Health program and projects can be found here.
4. Community Governance Community Governance: Have you built trust in your community around the use of data?
Establishing good governance frameworks
The use of data to address social problems will often entail important issues of governance and compliance. It is important to also put those issues in the context of the community of stakeholders with interests in the data and its use. Building strong relationships with that community will help promote good governance and identify new and permissible uses that can lead to unexpected benefits from a data collaboration or related initiative. When these opportunities are trusted by your community, they can amplify the benefits of your data initiative for all the involved or impacted organizations, individuals, and communities.
Good governance frameworks can help mitigate risks. These risks can be legal and regulatory, but risks to public trust and reputation are also of significant importance to organizations. These need to be balanced against the risks of not providing access to data for public interest purposes. The GovLab, and others, frame this process as finding ways to avoid both misuse, such as unauthorized uses that harm the data subjects, and missed uses, including failed opportunities to have improved people’s lives through the reuse of data. Below are examples of governance considerations that, when deliberated and applied in the early stages, can be used to achieve both risk mitigation and enhanced opportunities for data use.
Governance that engenders transparency and compliance
What if we could enable the use of data that everyone can understand? This could include developing an initiative with any of the following considerations built-in from the start:
- Transparency in the initiative’s governance and governance boards
- Transparency in the initiative’s purpose
- Transparency in the data collected, accessed, or created
- Transparency in how the use of the data complies with laws and regulations
- Identifying a data steward
- Securely storing and sharing data
- Providing access or sharing data in a manner that preserves privacy and commercially sensitive information
- Delivering insights to a broad range of stakeholders where benefits are shared
- Using approaches that give individuals and organizations a say in aspects of how data is collected, used, stored, and shared
Governance that creates community support
What if we could collect data that helps local communities solve local problems? What if we used data to address barriers to inclusion? Answers to questions such as these will help show the public that leveraging data in new and responsible ways is in their interest and can have positive effects over the long term.
Governance that enables open use
What mechanisms are in place to ensure that the data can be shared and used? Considerations may include:
- How to make the data “as open as possible” to aid innovation and use and combine the data in new and interesting ways. Can the data be made open or shared in a trusted manner, such as through anonymization?
- How can the data be made interoperable using common data models, standards, or stable identifiers? For example, the FAIR Principles provide guidance for improving the Findability, Accessibility, Interoperability, and Reusability of research data.
Mapping out these objectives can be very helpful in developing a governance framework for a fair, open, and trustworthy data ecosystem. A useful tool for developing and evaluating how data is used is the Open Data Institute’s Data Ethics Canvas.
Throughout the lifecycle of the initiative, the governance framework should be revisited to create a feedback loop to ensure that objectives are being constantly reassessed, particularly if those objectives change. You may decide that governance decisions are best delegated to a group of stakeholders or an independent body, for example to a governance board or an independent data steward. When decisions are delegated, it can be particularly important to have guidelines or an agreed principled approach that will enable those decisions to develop in a way that is in the spirit of the initiative.
Additionally, the co-creation of principles and conditions under which data is accessed and re-used through direct deliberations, such as the model presented by The GovLab’s The Data Assembly, may provide for an additional social license for data collaboration.
A principled approach
A principled approach to governance will help you develop a governance framework that goes beyond legal and compliance considerations. This can be helpful when multiple organizations are involved in a data innovation initiative. By initially agreeing on the principles by which you want to collect, store, use, and share data, the group will be empowered to make decisions in the future. The principles may in some cases be enshrined in a data charter.
As a starting point to assess and evaluate your policies and principles for sharing data, Microsoft published five principles that inform our contributions and commitments to trusted data collaboration. We hope these principles will inform the broader conversation on open data and that others can build on and improve them. The five principles are:
- Open – We will work to make data that is relevant to important social problems as open as possible, including by contributing open data ourselves
- Usable – We will invest in creating new technologies and tools, governance mechanisms and policies to make data more usable for everyone
- Empowering – We will help organizations generate value from their data according to their choices, and develop their AI talent to use data effectively and independently
- Secure – We will employ security controls to ensure data collaboration is operationally secure where it is desired
- Private – We will help organizations to protect individuals’ privacy in data sharing collaborations that involve personally identifiable information
Profile: The Public-Private Data Partnership Underway in London
An example of community governance is the Data Charter published in 2021, following the recommendations of the London Data Commission.
Unlocking data-led solutions is critical to solving issues affecting the city’s future growth. Without synergy between local authorities and private interests, finding solutions to pressing problems like improving air quality, shortening commuter times, improving transit, and reducing congestion would be impossible. In late 2019, the business group London First convened a group of public and private organizations as members of the London Data Commission. The Data Commission, steered by a project team of delivery partners, including London First, Arup, Oliver Wyman Forum, and Microsoft, brought local authorities and private companies together around sharing data as openly as possible. The Data Commission was tasked to serve as the authoritative business voice on city data and to help launch a data sharing ecosystem by creating data quality standards and addressing issues like privacy, ethics, and trust. In September 2020, the London Data Commission developed proposals for a Data for London framework. This framework recommended the delivery of a London Data Board and a London Data Charter.
Following the recommendations of the London Data Commission, London First created a working group to deliver on these recommendations and continue to work with the Chief Digital Officer for London to deliver the London Data Board and develop the London Data Charter.
The London Data Charter is built on a seven-principle framework: Deliver benefit for Londoners; Drive inclusive innovation; Protect privacy and security; Promote trust; Share learnings with others; Create scalable and sustainable solutions; and Be as open as possible. A broad range of companies have committed to the framework, and the charter is now considering milestones for London in how it works with the city’s business community to collaborate in securing data for the benefit of public projects.
Learn more about the London Data Commission here, and the London Data Charter here.
5. Technology and Data Technology and Data: Which solutions and resources do you need to measure, enable, and enhance your impact?
Factors for determining the right technical infrastructure
Fundamental to open data is the technical infrastructure needed to work with that data and support data sharing. This includes data analytics and data visualization tools, as well as technologies and platforms to access and share data within and across organizations easily and securely.
To determine the technological and platform needs of your data initiative, important factors to consider include:
- Is the data sensitive?
- What do you need to protect, and to what standard legally or contractually?
- What should you protect, taking into account ethical, reputational, and commercial considerations?
- For what purpose is the data being made available?
- To whom is the data being made available?
- What level of utility is required to work with the data?
- Can the requisite level of utility be achieved by applying privacy enhancing technologies?
- What platforms exist to facilitate data sharing and access, in accordance with required standards?
- For sensitive data, what governance frameworks are in place to control access and sharing?
The GovLab’s Data Responsibility Journey is an assessment tool that seeks to enable a review of such questions in an interactive manner.
Each of these factors may point to a range of technological needs. For example, data analytics tools can help to track trends, identify problems and efficiencies, as well as make predictions. Data visualization tools allow you to visualize the data you’re working with, and visually manipulate it.
In scenarios where privacy must be protected, a range of privacy-preserving techniques should be considered, such as anonymization and de-identification. Differential privacy is an industry-driven technique to enable data to be made more open in a way that does not put data protection at risk. Conceptually, differential privacy uses two steps to achieve privacy benefits:
- First, noise is added to each result to mask the contribution of individual data points. The noise is significant enough to protect the privacy of an individual, but with the aim to not materially impact the accuracy of the answers extracted by analysts and researchers.
- Secondly, the amount of information revealed from each query is calculated and deducted from an overall privacy-loss budget. Once the privacy budget is fully used, the data is retired and no additional queries are allowed to avoid any personal privacy compromise. This can be thought of as a built-in shutoff switch that prevents the system from showing data when it may begin compromising someone’s privacy.
When it comes to security, it’s important to consider the policy or state of the data lifecycle that is meant to be enforced, as well as the right mechanisms to achieve your organization’s security goals. Controlling access to data and ensuring those granted access are authorized and properly authenticated is critical, but additional technical measures may be needed depending on the data and how it is to be used. Confidential computing helps protect sensitive data in the cloud by offering security through data-in-use encryption that provides additional protection for your data while it’s being processed and enables greater collaboration across organizations.
Profile: How Privacy Enhancing Technologies Helped to Assess the Impact of Remote Learning on Young Students’ Education
Eight months into the COVID-19 pandemic, the Open Data Institute and Microsoft initiated an Education Open Data Challenge to look at the impact of the transition to remote learning on young students’ education.
To give challenge participants access to new and relevant datasets, Microsoft published United States Broadband Usage Percentage Datasets, both at a county level and at a ZIP code level, derived from anonymized data we collect as part of our ongoing work to improve the performance and security of our software and services. The ZIP code level dataset provides a granular view of broadband usage percentages by households within a ZIP code, so we took an additional step to ensure data privacy guarantees. We applied differential privacy, adding noise to the data aggregations. BroadbandNow also participated, making its county-level pricing and broadband provider data available for the first time.
The Education Open Data Challenge yielded insightful submissions and analyses with combinations and visualizations of data. The challenge also served to highlight how more open data can be made available, while protecting privacy.
Read more about the Education Open Data Challenge.
Resources Leadership
Data Stewards Academy
For leaders who are seeking to use data for social innovation, the Open Data Policy Lab’s Data Stewards Academy: Developing a Data Reuse Strategy for Solving Public Problems provides a self-directed learning program.
Data Maturity Assessment
Social sector organizations can use data.org’s Data Maturity Assessment tool to help measure and understand where your organization stands today.
Opportunity
Data Ecosystem Mapping
For a Data Ecosystem Mapping exercise, visit the Open Data Institute’s Data Ecosystem Mapping: Tool and Guidance.
Data Landscape Playbook
For more on assessing the data landscape and the context in which your data initiative operates, including identifying the problem your initiative is seeking to address, visit the Open Data Institute’s Data Landscape Playbook.
Talent
Training Programs
When it comes to training programs to upskill current talent or sharpen the skills of existing talent, there are a variety of resources to draw from:
- LinkedIn Learning and Microsoft Learn courses for data analysts. LinkedIn Learning’s self-paced courses are taught by industry experts, and the Microsoft Learn courses offer short step-by-step tutorials, browser-based interactive coding and scripting environments, and task-based achievements.
- Microsoft Certifications on data and AI fundamentals. Industry-recognized Microsoft Certifications help talent validate their skills and ability to perform in a role using Microsoft technologies.
- Microsoft Digital Skills Center for Nonprofits. A collaboration between TechSoup Courses and Microsoft, specifically for nonprofits, to access Microsoft product training, including courses focused on Excel, Power BI, and more.
- Microsoft workshops and training sessions available via the Microsoft Store. These free, live training courses for business and professionals include introductory and deeper dive sessions.
- Microsoft Viva Learning. On-demand training is available as part of Microsoft Viva Learning in Microsoft Teams.
- MySkills4Afrika Initiative. Through MySkills4Afrika, Microsoft employees from around the world volunteer their time, talent, and expertise to help support individuals and organizations across Africa.
Data Skills Framework
For additional resources, refer to the Open Data Institute’s Data Skills Framework.
Governance
Data Ethics Canvas
The Open Data Institute’s Data Ethics Canvas is a useful tool for developing and evaluating how data is used.
The Data Assembly
The GovLab’s The Data Assembly provides a model for the co-creation of principles and conditions under which data is accessed and re-used through direct deliberations.
London Data Charter
The London Data Charter, published in late 2021, is an example of community governance in-action.
Technology and Data
Data Responsibility Journey
When assessing an organization’s technology and resource needs for a data collaboration or initiative, The GovLab’s Data Responsibility Journey is a tool that outlines the opportunities and risks to consider at each stage of the data lifecycle.
SmartNoise
Microsoft’s collaboration with the OpenDP Initiative, led by Harvard, released SmartNoise, a first-of-its-kind open source platform for differential privacy. Anyone can begin utilizing the platform to make their datasets widely available to others around the world. The open source code and examples are available on GitHub.
Confidential computing
Confidential computing helps protect sensitive data in the cloud by offering security through data-in-use encryption that provides additional protection for your data while it’s being processed. A helpful introduction to confidential computing can be found here, along with example projects here.
Azure Data Share
Microsoft has several technologies that support more open data across a variety of use cases, such as Azure Data Share, which enables organizations to simply and securely share data with multiple customers and partners and provides the ability for organizations to combine internal data with partner data for new insights.
GitHub
GitHub is the world’s largest software development and code hosting platform. GitHub is frequently used for data projects, particularly for smaller datasets, collaboratively versioned data, data co-located with code, and machine learning workflows. GitHub supports rendering data and notebooks in various formats.